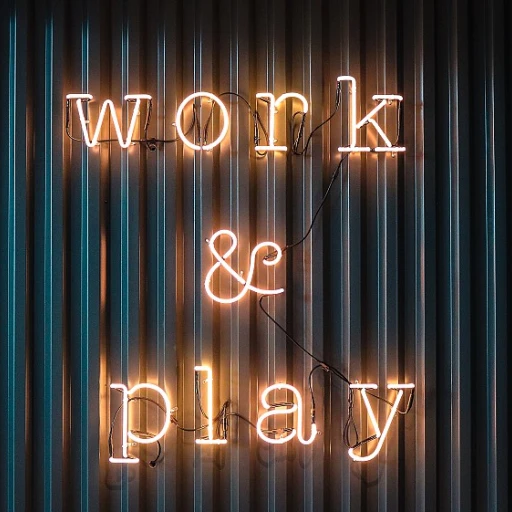
The Backbone of Decision-Making: Data at the Helm of Strategy
Steering Companies with Data-Driven Insights
Imagine a ship navigating the tricky waters of the business sea, where amidst the fog of competition, the captain—a company's strategic decision-maker—relies on a compass, sextant, and charts to plot the course. In today's scenario, that navigational toolkit is data. The backbone of decision-making is no longer gut feeling or intuition; it is solid, quantitative analysis that drives enterprises towards success. In fact, McKinsey's research suggests that data-driven organizations are 23 times more likely to acquire customers and 6 times as likely to retain them. This isn't just conjecture; it's the reality of modern business.
Charting a Course with Confidence
Data's role in strategic planning is akin to the cartographers of the past, creating maps that accurately represent the business landscape. From internal metrics like employee productivity to external factors such as market trends, data pieces together a picture of where a company is and where it needs to go. For instance, an article in Harvard Business Review notes how experiments and analytics can unlock understanding that drives impactful strategic decisions. Well-executed data analysis provides the confidence needed to make bold decisions—such as entering a new market or pivoting a product line with conviction.
Sailing the Seas of Data Maturity
The journey to becoming a data-informed organization isn't without challenges. An MIT Sloan Management Review study indicates that less than 20% of surveyed companies can be considered data-savvy. Yet, those who climb the data maturity ladder are found to be more competitive and agile. This points to a clear correlation: the depth of data integration within company strategies is directly linked to performance outcomes against competitors who may be slower in adopting data practices.
The New Chart-Makers: Role of Data Scientists and Analysts
Enter the data scientist—the modern chart-maker, a role that didn't exist in most companies a couple of decades ago. The proliferation of data has birthed this new profession, one that Payscale reports commands an average salary often in the six-figure range. The surge in demand for analysts and scientists signals a transformation: companies are investing heavily in personnel who can not only collect and clean data but can also discern patterns and insights that guide strategic voyages. Similarly, the machine learning algorithms they create are like the automated sails that help propel the ship forward, adjusting to the ever-shifting winds of business trends.
Translating Data into Currency: The Profit-Generating Power of Analytics
The Transformation from Raw Data to Business Gold
In an era where every click, swipe, and customer interaction is captured, the alchemy of turning raw data into actionable insights has revolutionized the business battleground. Companies today aren't starving for data; they're parched for analysis. In this regard, businesses that excel in data analytics are those mastering the art of refining information into strategy. It's not just about having data—it's about understanding how to use it to drive profit and innovation.
For instance, a study by the International Institute for Analytics noted that businesses utilizing data-driven approaches could see a 15-20% increase in revenue. What's more telling is a McKinsey Global Institute report which highlighted that high-performing data-driven organizations are 23 times more likely to acquire customers than their peers.
Spotlight on Industry Leaders
Take, for example, streaming giants who tap into the veins of customer preferences with analytics. They don't just guess what viewers might like; they employ sophisticated machine learning algorithms that tailor recommendations based on past behavior, trending topics, and similar user profiles. By leveraging analytics and data science, they've turned user data into a predictable revenue stream, cultivating customer loyalty as they do.
Leading tech firms, on the other hand, analyze vast datasets to forecast market needs, shaping their product roadmaps with a precision that often makes it seem like they have a finger on the pulse of the future. They're not fortune-tellers but astute analysts of patterns and trends.
The Nuts and Bolts of Profitable Insights
Behind this profitable use of data is, often, an army of skilled data scientists and analysts. According to Statistics, the demand for these professions is expected to surge by 28% by 2026. These roles are no longer confined to Silicon Valley; they spread across industries as varied as finance, health care, and retail. Analysts are the new-age prospectors finding veins of gold in the form of actionable insights.
Learning programs are catching up too. University campuses worldwide are reshaping their curricula, offering graduate programs and certifications in data analytics and business analytics. Post-graduate programs with a focus on analytics data science have become particularly attractive, promising a lucrative ROI for future data professionals.
Animating Numbers into Business Strategies
It's not just about crunching numbers; it's about telling a story that resonates with stakeholders. Hence, sophisticated data visualization tools have become crucial in translating obscure datasets into clear, actionable business strategies. Companies are investing in technologies and platforms where data analysis becomes not just accessible but also intuitive.
The importance of visual storytelling in data is underscored by a Forrester report stating that up to 70% of business users could adopt data visualization tools by 2025. Potent visual insights can lead to quicker strategic decisions and a deeper understanding of business challenges and opportunities.
Conclusively, as the bridge between data and profitability strengthens, the business landscape continues to evolve. It's a narrative of transformation, where analytics and data science are prime protagonists turning the wheels of commerce with precision and foresight.
From Data Skepticism to Advocacy: Cultivating an Analytic Mindset in Leadership
Embracing the Data-Driven Shift in Corporate Culture
In the not-too-distant past, boardrooms buzzed with gut feelings and 'tried-and-true' methods. Today, however, there's a seismic shift towards a more analytics-savvy leadership. Renowned experts like Thomas H. Davenport, author of 'Competing on Analytics', argue that the competitive edge lies in an analytic mindset. Indeed, businesses are recognizing that data science and analytics are not just back-office functions; they are strategic imperatives essential for top-level decision-making.
To illustrate, a Payscale survey highlights that over 50% of companies consider data analysis a key skill for their executives. Schools are responding, with programs such as MIT's Sloan School of Management integrating data analytics into their leadership curriculum. One such practitioner, a data scientist turned CEO, recently cited that integrating data insights fundamentally realigns market approach, boosting sales by 30% within a quarter.
Nurturing Analytical Acumen in Leadership Circles
Changing the mindset starts with advocacy—from within. Forward-thinking leaders are now acting as analytics champions. Educational platforms, like SAS's e-learning models, have become instrumental in cultivating these skills. AWS's reinforcement of cloud analytics highlights the corporate shift towards data utilization in executive training. Reports from the business analytics realm indicate that companies with strong analytical leadership see profit margins swell by 8% above their competitors, as per a study by Deloitte.
Tales of transformation from SVM (statistical vector machines) sceptics to data-driven decision-makers within the C-suite underscore the importance of a data-foundation. For instance, a long-term IBM analysis revealed that companies emphasizing analytics in leadership saw a notable improvement in driving their strategic initiatives.
Learning from the Ground Up: Structured Pathways to Analytic Proficiency
Creating a culture of data advocacy doesn't happen overnight. It requires deliberate learning pathways from analytics university programs to in-house training sessions. Google's analytics academy has set a benchmark by offering accessible courses for budding analysts and seasoned executives alike. Stanford University, on the other hand, showcases a progressive approach with their blend of machine learning and statistical analysis within their graduate program data science offerings.
Additionally, we see a rise in organizations investing in personalized data analytics training programs. There's an increasing trend of workshops that demonstrate real-time analytics applications, driving the point home that analytics and data science are the bedrock of modern strategic planning.
Unlocking Market Trends: Predictive Analytics as Your Crystal Ball
The Role of Predictive Analytics in Strategy
Imagine the edge a business can gain when it accurately foresees market trends and customer behavior. That's where predictive analytics comes into play. This analytical superpower utilizes historical data, statistical algorithms, and machine learning techniques to identify the likelihood of future outcomes. According to a recent report by Gartner, businesses leveraging predictive analytics can anticipate customer needs with a startling accuracy of up to 95%.
Analytics and data science have transformed passive data repositories into dynamic predictive tools, giving companies the ability to turn insights into action before trends hit the mainstream. Leading corporations utilize these insights to position products, optimize supply chains, and shape customer experiences. For instance, Amazon's anticipatory shipping model, which uses predictive data to streamline deliveries, exemplifies this approach's effectiveness.
Case Studies of Predictive Analytics in Action
A closer look at industry leaders such as Netflix and Starbucks reveals the profound impact predictive analytics has on strategy. Netflix's recommendation system, built on machine learning algorithms, not only enhances user experience but also drives content creation strategies, resulting in high audience retention rates. Similarly, Starbucks employs predictive models to determine ideal store locations, and through their rewards program, they personalize marketing strategies leading to increased customer loyalty.
These examples represent a broader trend highlighted in a study by the International Institute for Analytics, which found that businesses that employ predictive analytics in their operations can outperform competitors by generating 73% higher revenue.
Addressing the Misconceptions Around Predictive Analytics
Despite the considerable advantages, misconceptions about predictive analytics exist, such as overestimating its complexity or underutilizing its potential. As reported by Forbes, a common pitfall is neglecting the human element — data scientists must collaborate closely with business strategists to translate technical data into actionable business insights. Effective communication of analytics fosters informed decision-making and devises strategies with a real competitive edge.
Recognizing its transformative capability, Harvard Business School includes predictive analytics as a core topic in its MBA program, emphasizing the symbiotic relationship between analytics and business acumen. This approach prepares a new cadre of professionals who can navigate the data analytics landscape effectively.
Beyond the Numbers: Marrying Data Science with Human Intuition in Strategy
Integrating Instinct with Information
When it comes to carving out a competitive edge, it's not just about the cold, hard data—it's also about the warm touch of human insight. Data scientists are akin to the modern soothsayers of business strategy, but their predictions and models are most potent when paired with the seasoned instincts of business veterans. One studies shows that companies effectively combining analytics with managerial intuition can see an improvement in decision-making accuracy by up to 25%. Rich insights gleaned from data analysis become actionable when they resonate with a leader's experiential wisdom.
Case Studies in Strategic Synergy
Consider how leading tech companies harness data science analytics to drive innovation while trusting leadership’s instincts on when to pivot or persevere. There's a narrative to numbers that experienced professionals can read, much like a seasoned sailor can interpret the sea. A report by the Business Application Research Center underlined that data visualization tools could enhance intuition-driven decision-making, allowing managers to spot patterns and trends that machine algorithms might miss.
Expert-endorsed Approaches to Balanced Decision-Making
Experts in the field, such as Thomas H. Davenport, author of 'Competing on Analytics', argue for an approach where analytics and data science serve as indispensable co-pilots to a strategist’s intuition. Davenport's extensive work highlights that harnessing both aspects can lead to superior strategic outcomes. This dual approach is becoming a staple at universities offering graduate program data and business analytics courses focusing on the interplay between number-crunching and seasoned judgement.
Nurturing a Collaborative Ecosystem
The savvy business leaders are those who create an ecosystem where analysts and executives speak the same language. As data science university programs and educational platforms evolve, they instill a recognition of the value of this symbiosis in their curriculum. For instance, at the intersection of statistics and strategy, a notable trend is the rise of courses geared towards helping analysts augment their technical knowledge with strategic thinking skills. This kind of educational blend fosters a generation of professionals skilled in both analytics data science and intuitive strategy.
The Controversial Dance of Data and Intuition
Yet, it's not all smooth sailing. Some purists argue that strategy should be purely data-driven and that intuition can lead to bias and error. However, a counter-argument cites cases like Netflix's intuitive bet on producing original content, a move that data alone might not have fully supported at the time. These debates highlight the ongoing evolution in leveraging analytics and data science for strategic advantage. What is unmistakable is that the most advanced machine learning data systems today still benefit from the interpretative flair of their human operators.
Emerging Trends in Data-Informed Intuition
The budding synergy between data and intuition is giving rise to new management paradigms. Emerging trends suggest a push towards enhancing machine learning algorithms with inputs from domain experts, enriching predictive models with tacit knowledge that isn't easily quantifiable. This trend leverages the power of machine learning data while respecting the irreplaceable value of human foresight, a combination that is shaping the future of strategic planning in businesses worldwide.
The Educational Arms Race: Preparing the Future Workforce in Analytics and Data Science
Equipping Tomorrow's Leaders with Cutting-Edge Skills
In the arena of business strategy, the demand for professionals well-versed in analytics and data science is surging. As organizations strive to interpret the vast oceans of data they collect, the educational system is stepping up, churning out data scientists and business analysts equipped with the knowledge to transform data into actionable insights. Universities now offer specialized data science and analytics programs, blending theory with practical applications. For instance, the MIT Sloan School of Management integrates data science into its core curriculum, recognizing the pivotal role it plays in contemporary business strategies.
From Classroom to Boardroom: Real-World Applications
Graduates of data analytics programs are not just theory-heavy academics but poised professionals, brimming with case studies and hands-on experience. They transition seamlessly from the world of statistical analysis and machine learning algorithms in academia to applying these skills in the market. Notably, Harvard Business School alumni often recount their experience of utilizing data-driven strategies to optimize operations and drive growth within their companies.
Scaling New Heights: Data Skills in the Job Market
The value of a solid education in data science cannot be overstated when it comes to career prospects. According to a Payscale report, data scientists and data analysts rank among the most sought-after professionals in the job market, with their expertise in Python, SQL, and machine learning commanding competitive salaries. The transition from a college arts sciences background to a role where data analysis and data visualization skills are at the forefront signifies a shift in the caliber of expertise companies are actively seeking.
Nurturing the Next Generation of Data Pioneers
On the cutting-edge of this educational evolution are institutions like Stanford University and the University of California, Berkeley, which have developed programs that walk students through from the basics of computer science to the complexities of big data and IoT. Additionally, some schools even offer post-graduate programs with a focus on business analytics, positioning their graduates to be the architects of data-driven business strategies.
Ensuring a Diverse and Dynamic Talent Pool
It's heartening to see the efforts of universities to cultivate a varied talent pool in data science and analytics. Programs are increasingly designed to be inclusive, providing opportunities to a broad demographic of students who bring diverse perspectives to analytical challenges. This shift is not only a step towards democratizing the field of data science, but it also enriches the business landscape with a kaleidoscope of insights and innovation.
The Industry-Academia Nexus: Collaboration for Progress
Forging strong ties between the corporate sphere and academia is vital. Industry partnerships with universities allow for a cross-pollination of ideas and real-world testing grounds for burgeoning data analysts and scientists. Take for example SAS's collaborations with universities to integrate its analytics software into coursework, giving students a practical and valuable toolset that's directly applicable in business contexts.
Unclogging Bottlenecks with Automation: Machine Learning in Strategic Management
Streamlining Strategy with Machine Learning Enhancements
Machine learning has become the savvy strategist’s lever for prying open stubborn operational bottlenecks. Where human analysis might falter, the rhythmic hum of algorithms and models offers precision. Take, for instance, a case where data scientists turned to machine learning to decipher intricate patterns in customer purchasing behavior, leading to a more efficient inventory management and a noteworthy uptick in sales.
Case Studies: Machine Learning's Strategic Triumphs
Real-world examples, such as the transformation of supply chain management at leading retailers, validate the potency of machine learning in strategy. Analysts harnessed predictive models to adjust stock levels dynamically, avoiding overstocking woes and under-supply issues—this is analytics at its finest, creating robust systems that don't just react but anticipate.
Experts Weigh In on Machine Learning's Role in Strategy
Names like Andrew Ng, co-founder of Google Brain, and Cassie Kozyrkov, Chief Decision Scientist at Google, often surface when discussing the transformative impact of machine learning in strategic management. Their insights, coupled with studies from institutions like Stanford and MIT, highlight a clear trend: machine learning is not just an operational tool; it’s a strategic accelerant.
Automating Away the Monotony
Tasks that once tied up valuable human resources—from analyzing market data to gauging customer satisfaction—can now be automated, unlocking human potential to focus on the more creative aspects of strategy. The relentless churn of machine learning algorithms ensures that companies can keep their finger on the pulse of market dynamics with minimal human interference.
The Flip Side: Managing Machine Learning Implementations
However, it's not all smooth sailing. Automating strategy through machine learning presents its set of challenges, as indicated by anecdotes from industry veterans. The integration of complex algorithms into existing systems can be a grueling undertaking, one that necessitates carefully plotted roadmaps and a workforce skilled in both data analysis and interpretation.
Statistics Tell the Tale of Transformation
Conclusive figures underscore the impact of machine learning on company strategy. A PwC report concludes that AI and machine learning could contribute up to $15.7 trillion to the global economy by 2030. Specifically, in the domain of strategic management, automating data-related tasks has led to decision-making that’s not just faster but exponentially more informed.
Building a Future-Proof Strategy with Machine Learning
A concerted effort to embed machine learning into strategic management processes pays dividends by carving pathways through data mountains more efficiently and with greater acuity than humanly possible. As companies and universities collaborate to develop curricula that blend business acumen with analytics aptitude, the corporate world is maneuvering towards a future where automation and human expertise converge to create enlightened, data-driven strategies.
Measuring Mastery: Metrics and KPIs Guiding the Successful Implementation of Analytics
Grasping the Gauges: The Art of Picking Meaningful Metrics
In today's data-driven world, every boardroom echoes with the buzzwords of analytics and data science. However, the true marker of success isn't just the accumulation of data, it's the skillful application of relevant metrics and Key Performance Indicators (KPIs) that drive informed decisions and strategies. It's a delicate dance with numbers that goes beyond mere reporting, offering a magnifying glass to scrutinize the effectiveness of analytics and business tactics.
Anchoring Analytics with Actionable KPIs
Moving from theory to practice, companies looking to leverage analytics data for strategic gains must settle on KPIs that align with their unique business goals. These indicators act as navigational stars, guiding firms through the competitive terrain. For instance, a data analyst at a tech startup might focus on user engagement metrics, while their counterpart in retail analyzes customer churn rates. It's a contextual approach that imbues numbers with meaning, making data a storyteller rather than a silent statistic.
Deriving Dynamic Insights from Static Statistics
Amidst the terabytes of data, data scientists and analysts are the unsung heroes, weaving narratives that propel businesses forward. Consider how machine learning models transform static user data into predictions of buying behavior, offering a peek into future trends. These foresights, grounded in machine learning data, become invaluable when paired with strategic planning, turning dry stats into actionable insights.
Case Studies: Celebrating Wins with Data
Peering into the successes of leading corporations, one can witness the triumph of a well-oiled data strategy. Take, for instance, a global e-commerce giant that harnesses machine learning algorithms to predict inventory needs, thus achieving unprecedented efficiency in logistics. Or a financial services firm employing advanced data analytics to reduce fraud by pinpointing abnormal patterns. These case studies exemplify how the precise application of data science can drive company strategy and deliver tangible outcomes.
Navigating the Nuances: The Continuous Evolution of KPIs
It's important to acknowledge the organic nature of KPIs. What serves a company today may not fit the frame of tomorrow. Adapting and evolving these metrics is the bread and butter of a nimble strategy. Analysts and data scientists need to be as dynamic as the data they decipher, ensuring that KPIs reflect the current business landscape. Through an ongoing dialogue with data, businesses remain agile and informed, ready to pivot with precision when the tides of industry shift.